[ad_1]
<div _ngcontent-c18 = "" innerhtml = "
In this article, I will tell you about automate business strategies using artificial intelligence, and observe some recent progress in the automatic generation of strategies in the face of uncertainty.
Every day, advances in artificial intelligence are about tasks that are currently done only by humans, and it is interesting to consider in the short term what all this means for your business.
Games like chess have been tackled with artificial intelligence with amazing results, but there was a big gap between these games – where everything about the state of play and its consequences is known before taking a decision – and the reality of life where, like poker, there are decision makers only have a little information, and the quality and quantity of information used to make decisions vary Verry much. We humans are faced with this situation of great uncertainty every time we cross the street or eat a hamburger, but that does not seem to bother us. Until recently, computers have a hard time managing games that provide the decision maker with incomplete information about the state of the game. In addition, developing real-time strategies is more difficult than deciding if you want to bet or go to bed. Strategic plans may include several steps that must be sequenced properly, and these plans may include contingency plans.
The future is already here – it is not distributed very evenly.
Artificial intelligence is very popular, and for good reason, but many sources of information on pop culture lose the "how" of all this and focus instead on the dream of what could happen in the distant future. vaporware is common in the industry. This is not a good situation as ordinary people do not see the connection between research and the resulting products and fear what they do not understand.
I am a practitioner in the field of artificial intelligence, and my problem with Fever Futurism is an endless focus on a distant future devoid of a simple and rational vision of reality in the world. here and now. the tyranny of the rocket equation has blocked the futuristic promises of spaceflight for a generation and advances in artificial intelligence are also limited by the inertia that any disruptive technology is facing. The fear of change brought about by advances in the field of artificial intelligence should not lead society directly to extreme solutions such as: universal basic income or strong regulation of artificial intelligence.
The artificial intelligence as it is developed today consists mainly of programming, data collection and mathematics. It is not bady and it works badly at first. Sometimes it does not work at all. The scary results you see in the demonstrations are the product of a lot of hard work to hide the weaknesses of a narrow machine intelligence. I'd like to think of the field of artificial intelligence as "Charlotte's Web", in that Wilbur (the artificial intelligence agent) retains all the information. attention of the show, but Charlotte (the programmer) stays up all night to turn the Web. When Wilbur gets all the attention at the fair, as expected, you have to wonder what the pig did to deserve that attention. Why did not the farmer sell a magic spider instead of a magic pig? Well, the short answer is that marketers want to promote pigs, but the deeper answer is that too few sources of information are looking behind the curtain to explain the cool demo motif, ruining the magic trick but illuminating the audience.
However, I do not want to give a hard time to the "real" futurists. Excellent book by Nick Bostrom "superintelligence"worth reading, and even just a watch of his TED Conference on Potential Future Hazards of Artificial Intelligence could change the way you think about these things. Real research is underway on the distant future of artificial intelligence, including uniqueness and artificial general intelligence, but this is simply not the case.
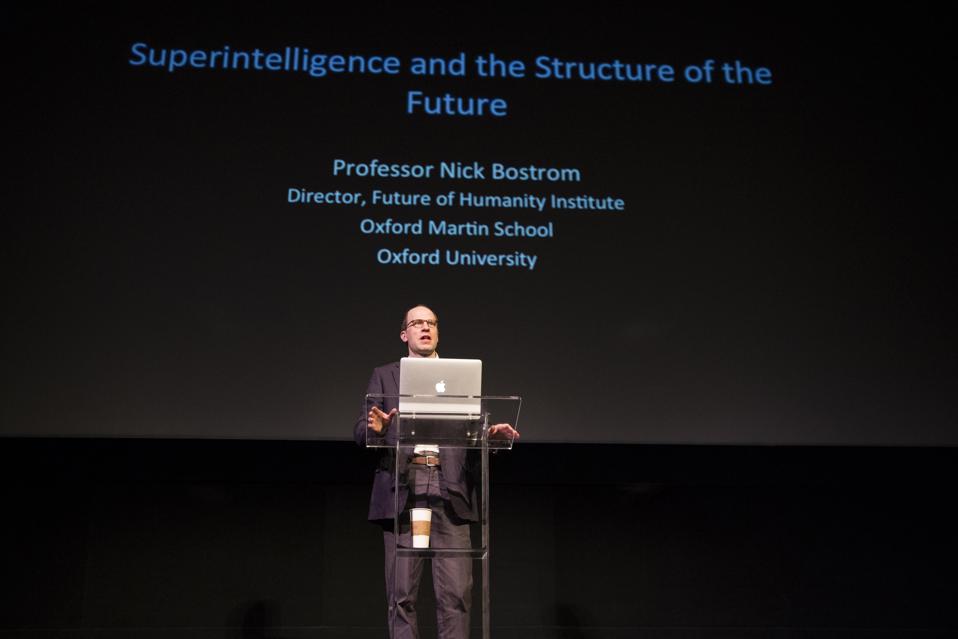
ASHEVILLE, NC – APRIL 24: Professor Nick Bostrom will be the keynote speaker at the Moogfest 2014 on April 24, 2014 in Asheville, North Carolina. (Photo by Alicia Funderburk / Getty Images)
Getty Images
relevant to your real life right now. It could be a day, but it is not today. What I want to share with you in this article is it here and now; The cruel reality of code compilation and deployment of solutions that companies use to earn money, save money and save time. Specifically, let's look at recent advances in strategic thinking developed by artificial intelligence. Yes, artificial intelligence is really applied to the decision-making model of governments and businesses. An example that comes to my mind immediately is a model of automatic learning of decision-making in China called the Policy Change Index.
Cool things are coming from the lab in the real world
I claim that the company should receive more meaningful information about what is happening currently in the field of artificial intelligence. For example, artificial intelligence agents are better at tackling difficult problems that they could not solve before, and these larger capabilities will be important. I'm talking specifically about AlphaStar from DeepMindand many similar systems that modernize the plumbing and brains that drive the global economy. This will have an impact on the way services are delivered because the strategy used in providing services has until recently relied on extremely heavy humans on keyboards wearing a helmet. It also has an impact on the financial sector, where 80% of the market activity is made up of algorithmic trading agents, and the automated strategy plays a major role in moving these agents to the markets.
AlphaStar from DeepMind at recently played online at StarCraft 2 in Europe., against human opponents who do not know that they play a computer. The core technology of DeepMind's approach to solving the theory of "imperfect information" games of the Real-Time Strategy (RTS) is a deep neural network formed from previous games and perfected by playing against himself to become even better. Personal play facilitates learning beyond simply copying decisions made in previous matches. The heart of the approach is a system that uses a mash-up of supervised learning and reinforcement learning, but the key concept to absorb is that a complicated RTS system reserved for humans is now solved very comprehensively by an artificial intelligence system. A predecessor of AlphaStar to play the game Go called AlphaGo. Unlike AlhpaGo, AlphaStar must develop and execute strategies based on an incomplete set of information. This is a big step forward, in a more realistic and realistic area.
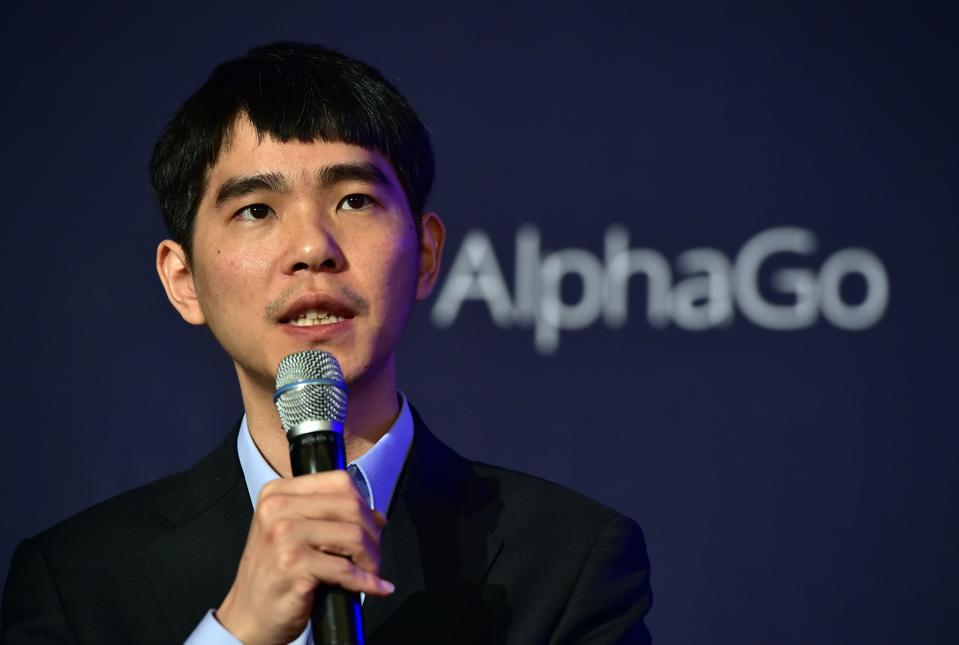
Lee Se-Dol, one of the greatest modern players in the old Go board game, will be speaking at a press conference after the third game of the Google DeepMind Challenge Match against the supercomputer developed by Google, AlphaGo, in a hotel in Seoul March 12
AFP / Getty Images
The first series of AlphaStar matches Earlier this year, they had opposed professional players in a controlled private setting, relying on their ability to perform incredibly high (superhuman) actions per minute to beat humans. Playing an information game as incomplete and difficult to play was really impressive, but it is not over yet.
Development agents are a bit like building a rocket and watching someone drive it. In the last few months since the news of AlphaStar, it has been said that the Casters watched AlphaStar play as programmers debugging their artificial intelligence agents: they look at the successes and they are watching. marvel at the ideas that the agent has proposed, and then look at the failures. and try to understand "Why did he do that?" Super bad error details in the game, such as Poor investment and monitoring strategies Then, badociate with super-low inactive details of the agent, such as badyzing the sequence of states that led the agent to learn how to do what. he did.
DeepMind is now trying to make AlphaStar even better by making it play under constraints that are more like those imposed on a human. DeepMind seeks to train agents such as AlphaStar to beat humans in tasks that humans are good at, and also strives to do it in the same way as humans, without resorting to game spam. nor to other unfair games. superhuman "cheats" that rely on being a computer to work. I am convinced that this is not the solution to all the problems that humans solve, but it is good that a subset of everything we do ourselves is to enter the realm of the world. 'automating. At present, the set of cards that AlphaStar can play seems still limited, but progress is underway, and we can see that this progress is progressing in real time. The initial demonstration of the system only played one of the three races StartCraft 2 (Protoss), but the most recent version works for the 3 races of the game. For me, this is the exciting part of the field of the game. Artificial intelligence today. Every day, the limit of what is possible increases, and there does not seem to be an end in sight.
Assuming that the aforementioned advances on generating strategies based on machine learning are at hand, what does an automated business strategy look like? From my experience, government and business projects contain limited scope requirements for modeling a part of the business strategy. Whether it's credit risk models, referral systems, customer segmentation, legislative risk models or trading algorithms, these limited-scope approaches have the advantage of being able to manage credit risk. 39 advantage of being easily summarized in a box of a flowchart with a name. Rather than global solutions that model an entire company, the most popular type of project today is a tool at the service of management. Predictive and decision-making tools are very popular right now, and I predict that at some point these little elephant bites will become global solutions. The repression I've heard and seen is that ERP systems promised to provide this kind of global strategy but did not, and that the technical debt In the current infrastructure, the best solution is to gradually change the way things work.
The automation of business strategy has been around for a long time, but with the recent advances in artificial intelligence, it should improve further. The ideas above should lead you to the inevitable conclusion that the development of the business strategy will benefit from greater automation, including artificial intelligence. Companies are organized in a certain way, with key performance indicators (KPIs) and KPI improvement plans. Expect artificial intelligence to introduce into business strategy discussions.
There is so much work to do
To build a system that generates strategies, a good starting point is to develop a simulator to define the boundaries of the world where the agent can play and make decisions. The simulator also needs a lot of data on previous decisions and their results to gain experience. This process of building a simulator also involves deciding which part of the world to simulate. DeepMind's approach to avoiding the big gap between beginner and novice agents was to teach the agent to mimic historical data and then rotate his game against copies of himself once on the right track.
Interpretability, data set processing tools, machine learning and prejudices are major obstacles to the widespread adoption of artificial intelligence for development and development. execution of autonomous business strategies.
Let's talk about interpretability first. We humans need to understand what the machine has learned from data, how it thinks and why it makes certain decisions. Researchers are now working to resolve these interpretability issues. Some recent progress interesting work on this topic is "A unified approach to interpret model predictions"and their open-source library form.
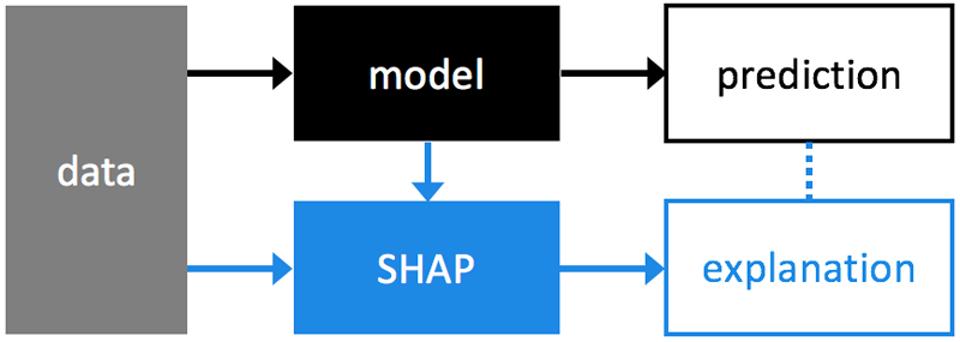
A unified approach to explain the outcome of any machine learning model.
Scott Lundberg
Beyond interpretability, another challenge is to manipulate data sets for the formation of these artificial intelligence agents and to tag the data without excessive human effort. Some important efforts in this direction should solve most of the problem. A remarkable project for the labeling, transformation and organization of datasets is snorkel.orgwhich has a large user base and support from the US government. Predefined datasets and templates are also becoming increasingly available as they are put into the public domain by researchers, companies, and governments adopting open data policies.
A third challenge, in addition to the interpretability and preparation of datasets, is the automation of the machine learning model building process, which often requires a computer scientist, a machine learning engineer and a DevOps engineer, as well as a project manager to ensure the smooth running of trains. time. There are many active automated machine learning projects such as Tpot, which aim to eliminate these humans from the process as much as possible. This automation is not yet a problem solved and gives you a realistic idea of what artificial intelligence researchers are shooting.
A fourth challenge that I want to bring to your attention is that of prejudices. Artificial intelligence systems are very effective at learning and perpetuating the biases that exist in the observed data. This is an important and unresolved area of machine learning that prevents some key business processes from easily adopting strategies generated with the aid of artificial intelligence. It is the process of verifying the absence of some bias in the generated tips that adds a significant cost to machine learning projects of this nature.
Rather than wait for all these problems to be solved, the world is progressing with the adoption of artificial intelligence on a large scale, and I think that the workings of the training will be slow, because these systems are becoming more and more more common in the company's infrastructure.
This article and those that follow should help you understand how the field of artificial intelligence is evolving and understand what all of this means for you right now. Let me briefly summarize the 5 main points to remember:
- Under certain constraints, artificial intelligence will be able to generate a business strategy
- The business strategy based on artificial intelligence will become more popular
- Do not panic. New things are happening, but Skynet is not just around the corner
- Computers become very good for tasks that only humans could do before, like driving
- Artificial intelligence, as a field of study, has not yet been invented. Humans are striving to define and solve many unresolved problems, both in the field of AI and as problems that AI could help solve.
">
In this article, I will tell you about automate business strategies using artificial intelligence, and observe some recent progress in the automatic generation of strategies in the face of uncertainty.
Every day, advances in artificial intelligence are about tasks that are currently done only by humans, and it is interesting to consider in the short term what all this means for your business.
Games like chess have been tackled with artificial intelligence with amazing results, but there was a big gap between these games – where everything about the state of play and its consequences is known before taking a decision – and the reality of life where, like poker, there are decision makers only have a little information, and the quality and quantity of information used to make decisions vary Verry much. We humans are faced with this situation of great uncertainty every time we cross the street or eat a hamburger, but that does not seem to bother us. Until recently, computers have a hard time managing games that provide the decision maker with incomplete information about the state of the game. In addition, developing real-time strategies is more difficult than deciding if you want to bet or go to bed. Strategic plans may include several steps that must be sequenced properly, and these plans may include contingency plans.
The future is already here – it is not distributed very evenly.
Artificial intelligence is very popular, and for good reason, but many sources of information on pop culture lose the "how" of all this and focus instead on the dream of what could happen in the distant future. The vaporware is common in the industry. This is not a good situation as ordinary people do not see the connection between research and the resulting products and fear what they do not understand.
I am a practitioner in the field of artificial intelligence, and my problem with Fever Futurism is an endless focus on a distant future devoid of a simple and rational vision of reality in the world. here and now. The tyranny of the rocket equation has held futuristic promises of spaceflight for a generation, and advances in artificial intelligence are also limited by the inertia that any disruptive technology faces. The fear of change brought about by advances in the field of artificial intelligence should not directly lead society to extreme solutions, such as universal basic income or strict regulations of artificial intelligence.
The artificial intelligence as it is developed today consists mainly of programming, data collection and mathematics. It is not bady and it works badly at first. Sometimes it does not work at all. The scary results you see in the demonstrations are the product of a lot of hard work to hide the weaknesses of a narrow machine intelligence. I'd like to think of the field of artificial intelligence as "Charlotte's Web", in that Wilbur (the artificial intelligence agent) retains all the information. attention of the show, but Charlotte (the programmer) stays up all night to turn the Web. When Wilbur gets all the attention at the fair, as expected, you have to wonder what the pig did to deserve that attention. Why did not the farmer sell a magic spider instead of a magic pig? Well, the short answer is that marketers want to promote pigs, but the deeper answer is that too few sources of information are looking behind the curtain to explain the cool demo motif, ruining the magic trick but illuminating the audience.
However, I do not want to give a hard time to the "real" futurists. Nick Bostrom's excellent book "Superintelligence" deserves to be read, and even a mere listen to his TED talk about the potential dangers of artificial intelligence could change your perception of these things. Real research is underway on the distant future of artificial intelligence, including uniqueness and artificial general intelligence, but this is simply not the case.
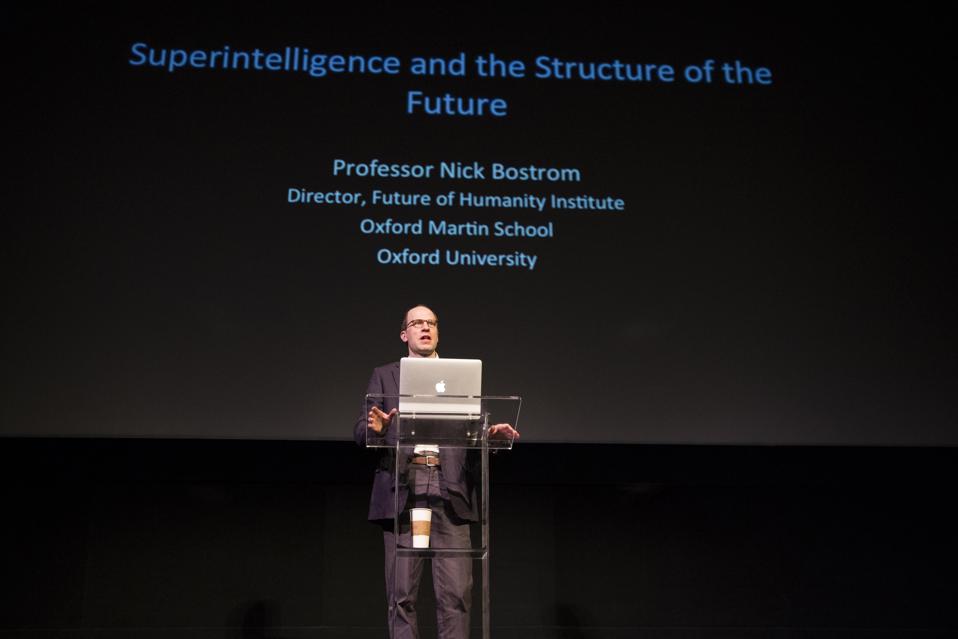
ASHEVILLE, NC – APRIL 24: Professor Nick Bostrom will be the keynote speaker at the Moogfest 2014 on April 24, 2014 in Asheville, North Carolina. (Photo by Alicia Funderburk / Getty Images)
Getty Images
relevant to your real life right now. It could be a day, but it is not today. What I want to share with you in this article is it here and now; The cruel reality of code compilation and deployment of solutions that companies use to earn money, save money and save time. Specifically, let's look at recent advances in strategic thinking developed by artificial intelligence. Yes, artificial intelligence is really applied to the decision-making model of governments and businesses. An example that immediately comes to my mind is a model of China's automatic decision-making process, called the Policy Change Index.
Cool things are coming from the lab in the real world
I claim that the company should receive more meaningful information about what is happening currently in the field of artificial intelligence. For example, artificial intelligence agents are better at tackling difficult problems that they could not solve before, and these larger capabilities will be important. I'm talking in particular about DeepMind's AlphaStar and many similar systems that modernize the plumbing and brains that run the global economy. This will have an impact on the way services are delivered because the strategy used in providing services has until recently relied on extremely heavy humans on keyboards wearing a helmet. This also has an impact on the financial sector, where 80% of the market activity is algorithmic trading agents, and the automated strategy plays a major role in moving these agents.
AlphaStar from DeepMind recently played in Europe at StarCraft 2 games online, against human opponents who do not know that they play on a computer. The core technology of DeepMind's approach to solving the theory of "imperfect information" games of the Real-Time Strategy (RTS) is a deep neural network formed from previous games and perfected by playing against himself to become even better. Personal play facilitates learning beyond simply copying decisions made in previous matches. The system is essentially based on a system of supervised learning and reinforcement learning, but the essential concept to remember is that a complicated RTS game for humans is being solved in a very complete way by a system of artificial intelligence. A predecessor of AlphaStar to play the game Go called AlphaGo. Unlike AlhpaGo, AlphaStar must develop and execute strategies based on an incomplete set of information. This is a big step forward, in a more realistic and realistic area.
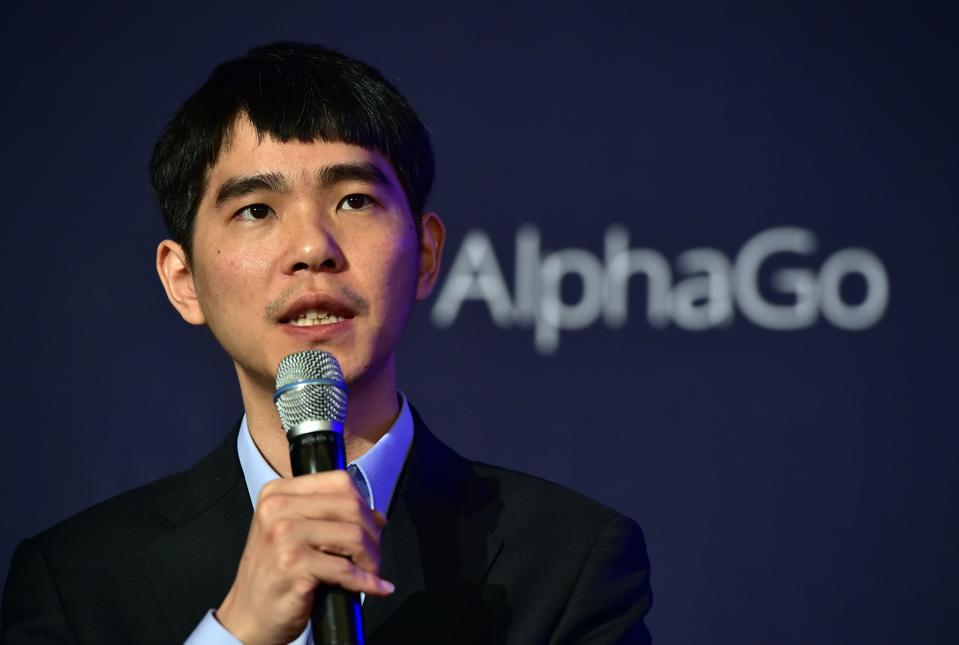
Lee Se-Dol, one of the greatest modern players in the old Go board game, will be speaking at a press conference after the third game of the Google DeepMind Challenge Match against the supercomputer developed by Google, AlphaGo, in a hotel in Seoul March 12
AFP / Getty Images
The first series of AlphaStar matches earlier this year was to confront professional players in a private and controlled environment, relying on the ability to perform an incredibly high (superhuman) number of games. actions per minute to beat humans. Playing an information game as incomplete and difficult to play was really impressive, but it is not over yet.
Development agents are a bit like building a rocket and watching someone drive it. In the last few months since the news of AlphaStar, it has been said that the Casters watched AlphaStar play as programmers debugging their artificial intelligence agents: they look at the successes and they are watching. marvel at the ideas that the agent has proposed, and then look at the failures. and try to understand "Why did he do that?" Super neglected error details in the game, such as misplacement strategies and screening strategies, and then a link with harmless details, such as badyzing the sequence of states that brought the agent to learn how to do what he did.
DeepMind is now trying to make AlphaStar even better by making it play under constraints that are more like those imposed on a human. DeepMind seeks to train agents such as AlphaStar to beat humans in tasks that humans are good at, and also strives to do it in the same way as humans, without resorting to game spam. nor to other unfair games. superhuman "cheats" that rely on being a computer to work. I am convinced that this is not the solution to all the problems that humans solve, but it is good that a subset of everything we do ourselves is to enter the realm of the world. 'automating. At present, the set of cards that AlphaStar can play seems still limited, but progress is underway, and we can see that this progress is progressing in real time. The initial demonstration of the system only played one of the three races StartCraft 2 (Protoss), but the most recent version works for the 3 races of the game. For me, this is the exciting part of the field of the game. Artificial intelligence today. Every day, the limit of what is possible increases, and there does not seem to be an end in sight.
Assuming that the aforementioned advances on generating strategies based on machine learning are at hand, what does an automated business strategy look like? From my experience, government and business projects contain limited scope requirements for modeling a part of the business strategy. Whether it's credit risk models, referral systems, customer segmentation, legislative risk models or trading algorithms, these limited-scope approaches have the advantage of being able to deal with risk. 39 advantage of being easily summarized in a box of a flowchart with a name. Rather than global solutions that model an entire company, the most popular type of project today is a tool at the service of management. Predictive and decision-making tools are very popular right now, and I predict that at some point these little elephant bites will become global solutions. What I have heard and seen is that ERP systems have promised to provide this kind of global strategy but have not, and that the technical debt in the current infrastructure is better supported by progressive changes in the functioning of things.
L'automatisation de la stratégie d'entreprise existe depuis longtemps, mais avec les progrès récents en intelligence artificielle, elle devrait s'améliorer encore. Les idées ci-dessus devraient vous amener à la conclusion inévitable que le développement de la stratégie d'entreprise bénéficiera d'une plus grande automatisation, notamment de l'intelligence artificielle. Les entreprises sont organisées d’une certaine manière, avec des indicateurs de performance clés (KPI) et des plans d’amélioration des KPI. Attendez-vous à ce que l'intelligence artificielle s'introduise dans les discussions sur la stratégie d'entreprise.
Il y a tellement de travail à faire
Pour construire un système qui génère des stratégies, un bon point de départ consiste à développer un simulateur pour définir les limites du monde où l'agent peut jouer et prendre des décisions. Le simulateur a également besoin de nombreuses données sur les décisions antérieures et leurs résultats pour acquérir de l'expérience. Ce processus de construction d'un simulateur implique également de décider quelle partie du monde simuler. L’approche suivie par DeepMind pour éviter le grand fossé entre les agents débutants et débutants consistait à apprendre à l’agent à imiter les données historiques, puis à faire pivoter son jeu contre des copies de lui-même une fois sur la bonne voie.
L'interprétabilité, les outils de traitement des ensembles de données, l'apprentissage automatique et les préjugés sont des obstacles majeurs à l'adoption généralisée de l'intelligence artificielle pour le développement et l'exécution de stratégies commerciales autonomes.
Parlons d'abord de l'interprétabilité. Nous, les humains, devons comprendre ce que la machine a appris des données, comment elle pense et pourquoi elle prend certaines décisions. Les chercheurs s’emploient maintenant à résoudre ces problèmes d’interprétabilité. Parmi les progrès récents, des travaux intéressants sur ce sujet sont "Une approche unifiée pour l’interprétation des prédictions de modèle" et leur structure de bibliothèque à source ouverte.
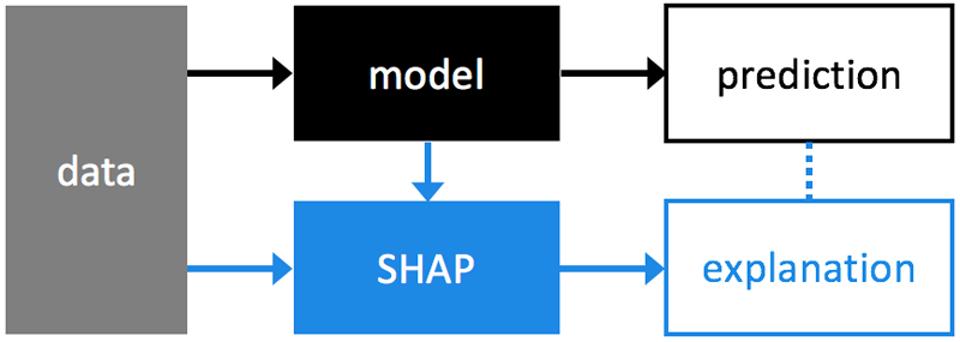
Une approche unifiée pour expliquer le résultat de n’importe quel modèle d’apprentissage automatique.
Scott Lundberg
Au-delà de l’interprétabilité, un autre défi consiste à manipuler des ensembles de données pour la formation de ces agents d’intelligence artificielle et pour étiqueter les données sans effort humain excessif. Certains efforts importants dans ce sens devraient résoudre la majeure partie du problème. Snorkel.org, qui compte de nombreux utilisateurs et bénéficie du soutien du gouvernement des États-Unis, constitue un projet digne d'étiquetage, de transformation et d'organisation des ensembles de données. Les ensembles de données et les modèles prédéfinis deviennent également de plus en plus disponibles, car ils sont mis dans le domaine public par les chercheurs, les entreprises et les gouvernements qui adoptent des politiques de données ouvertes.
Un troisième défi, en plus de l’interprétabilité et de la préparation des jeux de données, est l’automatisation du processus de construction de modèles d’apprentissage automatique, qui nécessite souvent un informaticien, un ingénieur en apprentissage automatique et un ingénieur DevOps, ainsi qu’un chef de projet pour badurer le bon fonctionnement des trains. temps. Il existe de nombreux projets d’apprentissage automatique automatisés actifs, tels que TPOT, qui visent à éliminer autant que possible ces humains du processus. Cette automatisation n’est pas encore un problème résolu et vous donne une idée réaliste de ce que les chercheurs en intelligence artificielle tirent.
Un quatrième défi que je veux porter à votre attention est celui des préjugés. Les systèmes d'intelligence artificielle sont très efficaces pour apprendre et perpétuer les biais qui existent dans les données observées. C'est un domaine important et non résolu de l'apprentissage automatique qui empêche certains processus métier clés d'adopter facilement des stratégies générées à l'aide de l'intelligence artificielle. C’est le processus de vérification de l’absence de certains biais dans les conseils générés qui ajoute un coût important aux projets d’apprentissage automatique de cette nature.
Plutôt que d’attendre que tous ces problèmes soient résolus, le monde progresse avec l’adoption de l’intelligence artificielle à grande échelle, et je pense que les rouages de la formation se feront lentement, car ces systèmes deviennent de plus en plus courants dans l’infrastructure de l’entreprise.
Cet article et ceux qui suivent devraient vous aider à comprendre comment évolue le domaine de l'intelligence artificielle et à comprendre ce que tout cela signifie pour vous en ce moment. Permettez-moi de résumer brièvement les 5 principaux points à retenir:
- Sous certaines contraintes, l'intelligence artificielle sera capable de générer une stratégie d'entreprise
- La stratégie d'entreprise conçue par l'intelligence artificielle va devenir plus populaire
- Ne panique pas. De nouvelles choses se pbadent, mais Skynet n'est pas juste au coin de la rue
- Les ordinateurs deviennent très bons pour des tâches que seuls les humains pouvaient faire auparavant, comme conduire
- L’intelligence artificielle, en tant que domaine d’étude, n’a pas encore été inventée. Les humains s'efforcent de définir et de résoudre de nombreux problèmes non résolus, à la fois dans le domaine de l'IA et en tant que problèmes que l'IA pourrait aider à résoudre.