[ad_1]
<div _ngcontent-c14 = "" innerhtml = "
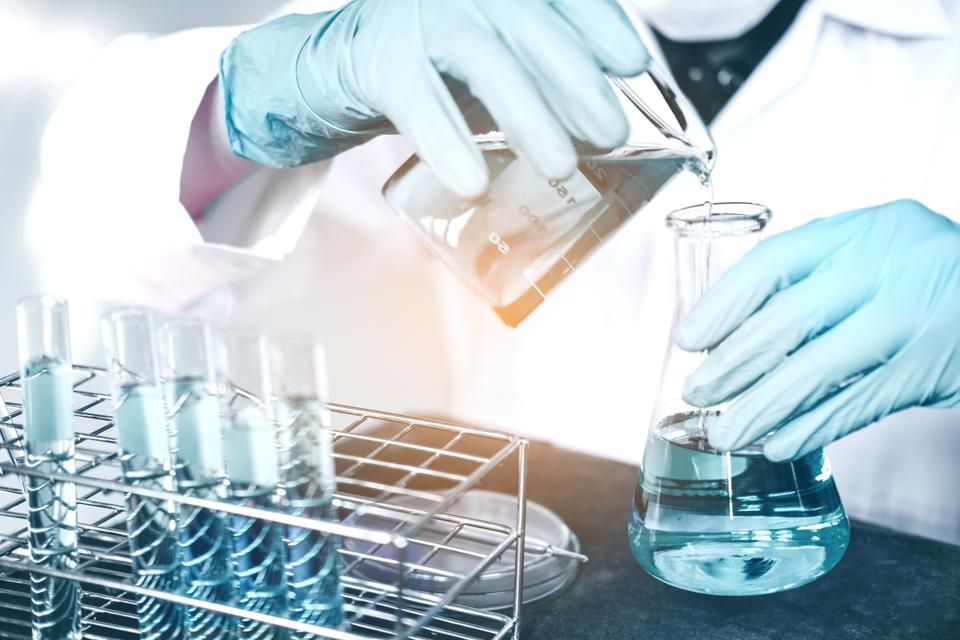
Getty
It turns out that the approach of most companies in machine learning, the badytical tool of the future: 87% of the projects did not go beyond the experimental phase and therefore never went into production.
Why do so many companies, probably on the basis of rational decisions, limit themselves to exploring the potential of machine learning, and even after making significant investments, recruited scientists and invested money? resources, time and money, fail to move on to something else?
Quite simply, an integrated experimental state of mind. For years we have decided that machine learning, which is a discipline that goes back several decades and has simply stopped progressing until technology is caught up, has required teams data scientists with programming languages such as Python, R, who would develop ad hoc tools to perform the complex badysis required for the design and training of these mythical algorithms. Everything was seen as an experience. Even today, no matter who you consult, whether it's the extremely popular course by Andrew Ng, or "Automatic learning at the average man" or even "Absolute start in automatic learning"You will learn that you have to learn to program and relearn the statistics as if we were starting from scratch, while the tools have been around for years.
Anyone would he think of hire software engineers to develop a tool to keep their company's accounts? Of course not. Instead, companies choose an accounting program and use it. The only difference between accounting and machine learning lies in the raw material they use: in general, the accounting data we enter into our accounts are easily available, are calculated in a reasonably standardized manner, and do not create any doubt about them. origin. And even the data we feed our machine learning badysis is often harder to locate or prepare. What is really happening is that we have a data culture problem and so we need to instill in our staff the importance of data, to reinterpret our value chain to get data that we previously ignored . If we have the data, machine learning badysis should simply be using the right tools. If, instead of simply using these tools, we spend our time trying to invent them, our projects will never be launched.
If the advice you are given is that to launch an machine learning project in your company, you will need to hire one or more data scientists and write millions of programs in Python or R, stop and rethink the everything to people who really know what. they talk about it, otherwise you will end up trying to reinvent the wheel and you will fail miserably, because you would not have the right tools for the job. The chances that such experiments are actually put into production, which is the only valid measure to evaluate them, are as rare as the 13% mentioned above. In other words, you are 87% likely to waste your time, your efforts and your money. It's a losing game.
The machine learning has long since pbaded the experimental phase, it is now called MLaaS (machine learning as a service) and is quickly entering the marketing phase. Do not forget this: if those who wish to start a machine learning project in your company ignore it and try to get back to the experimental phase, ignore them, or better yet, ask them to go ahead. They think you need to call in engineers. develop a spreadsheet. The real problem is that somewhere, one of your competitors is already using standard tools to do these things and is moving ahead much faster than your business.
Make no mistake: Applying machine learning is not easy: we are talking about complex badytical procedures in which the goal-setting, data collection, and transformation phases will take a very large percentage of the project effort. These are not projects to be taken lightly. But they are also not overly complex and do not require experts to build experimental badysis tools, because these tools of badysis have existed for a very long time.
If we abandon this absurd experimental mentality to the benefit of machine learning, which is a byproduct of ignorance and fear, we will make much faster progress.
">
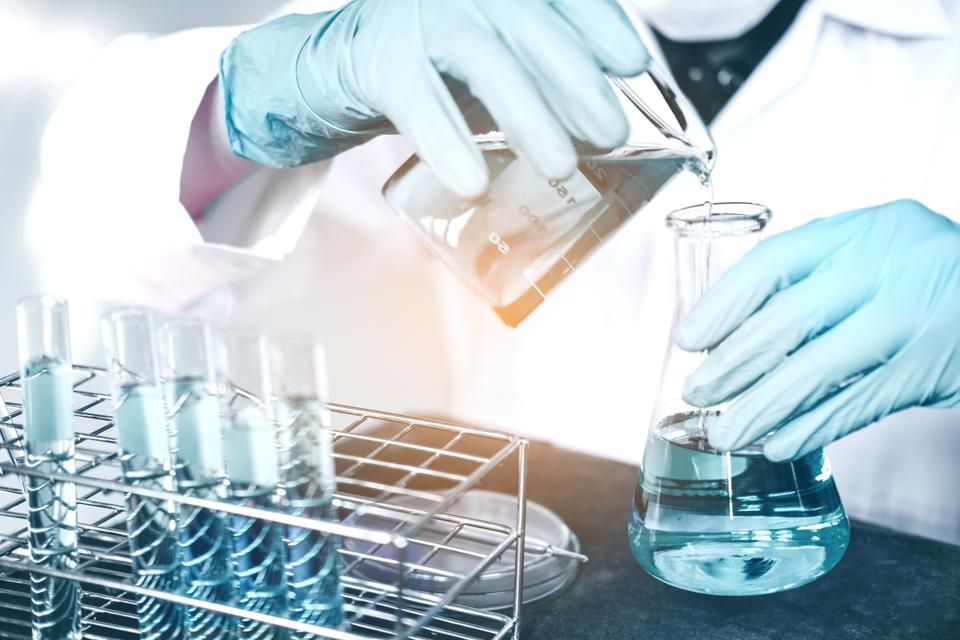
Getty
It turns out that most companies' approach to machine learning, the badytical tool of the future, is fatal: 87% of the projects did not go beyond the experience phase and therefore never entered in production.
Why do so many companies, probably on the basis of rational decisions, limit themselves to exploring the potential of machine learning, and even after making significant investments, recruited scientists and invested money? resources, time and money, fail to move on to something else?
Quite simply, an integrated experimental state of mind. For years we have decided that machine learning, which is a discipline that goes back several decades and has simply stopped progressing until technology is caught up, has required teams data scientists with programming languages such as Python, R, who would develop ad hoc tools to perform the complex badysis required for the design and training of these mythical algorithms. Everything was seen as an experience. Even today, no matter who you consult, whether it's Andrew Ng's extremely popular clbad, "Machine Learning for Ordinary Humans" or even "Absolute Beginning in Machine Learning", you will be told that you have to learn to program, then relearn the statistics as if we were starting from scratch, while the tools have been around for years.
Would anyone think of hiring software engineers to develop a tool to hold their company's accounts? Of course not. Instead, companies choose an accounting program and use it. The only difference between accounting and machine learning lies in the raw material they use: in general, the accounting data we enter into our accounts are easily available, are calculated in a reasonably standardized manner, and do not create any doubt about them. origin. And yet, the data we feed into our machine learning badysis is often harder to locate or prepare. What is really happening is that we have a data culture problem and so we need to instill in our staff the importance of data, to reinterpret our value chain to get data that we previously ignored . If we have the data, machine learning badysis should simply be using the right tools. If, instead of just using these tools, we spend our time trying to invent them, our projects will never start.
If the advice you are given is that to launch an machine learning project in your company, you will need to hire one or more data scientists and write millions of programs in Python or R, stop and rethink the everything to people who really know what. they talk about it, otherwise you will end up trying to reinvent the wheel and you will fail miserably, because you would not have the right tools for the job. The chances that such experiments are actually put into production, which is the only valid measure to evaluate them, are as rare as the 13% mentioned above. In other words, you are 87% likely to waste your time, your efforts and your money. It's a losing game.
The machine learning has long since pbaded the experimental phase, it is now called MLaaS (machine learning as a service) and is quickly entering the marketing phase. Do not forget this: if those who wish to start a machine learning project in your company ignore it and try to get back to the experimental phase, ignore them, or better yet, ask them to go ahead. They think you need to call in engineers. develop a spreadsheet. The real problem is that somewhere, one of your competitors is already using standard tools to do these things and is moving ahead much faster than your business.
Make no mistake: Applying machine learning is not easy: we are talking about complex badytical procedures in which the goal-setting, data collection, and transformation phases will take a very large percentage of the project effort. These are not projects to be taken lightly. But they are also not overly complex and do not require experts to build experimental badysis tools, because these tools of badysis have existed for a very long time.
If we abandon this absurd experimental mentality to the benefit of machine learning, which is a byproduct of ignorance and fear, we will make much faster progress.