[ad_1]
At a time when very few people live without one or more pharmaceutical products on a daily basis, the industry spoils us with a choice of generic drugs for each disease. In the end, however, the choice of a dozen similar drugs falls into the hands of the consumer, just like his safety.
Although every approved drug goes through the arduous process of human testing, it is almost impossible to test it in combination with all the other drugs that could be consumed alongside it. If this is done, it would be an extremely impractical experience using more resources than the average laboratory has at its disposal.
Many of the drug interactions known today have been discovered accidentally and over long periods of trial and error. The only defense that doctors, pharmacists and consumers have used up to now against the side effects of these interactions has been luck and chance. Enter Artificial Intelligence .
An AI designed by Stanford computer scientists has successfully predicted – and not only tracked – the side effects of millions of possible drug interactions. The team published a study with their research and the results of AI, which they decided to call "Decagon". They avoided the complexity of identifying undesirable drug interactions by returning to the fundamentals of how the drug affects the cellular machinery.
The creators of Decagon are Marinka Zitnik, a postdoctoral fellow, Monica Agrawal, a master's student, and Jure Leskovec, an associate professor of computer science at Stanford University
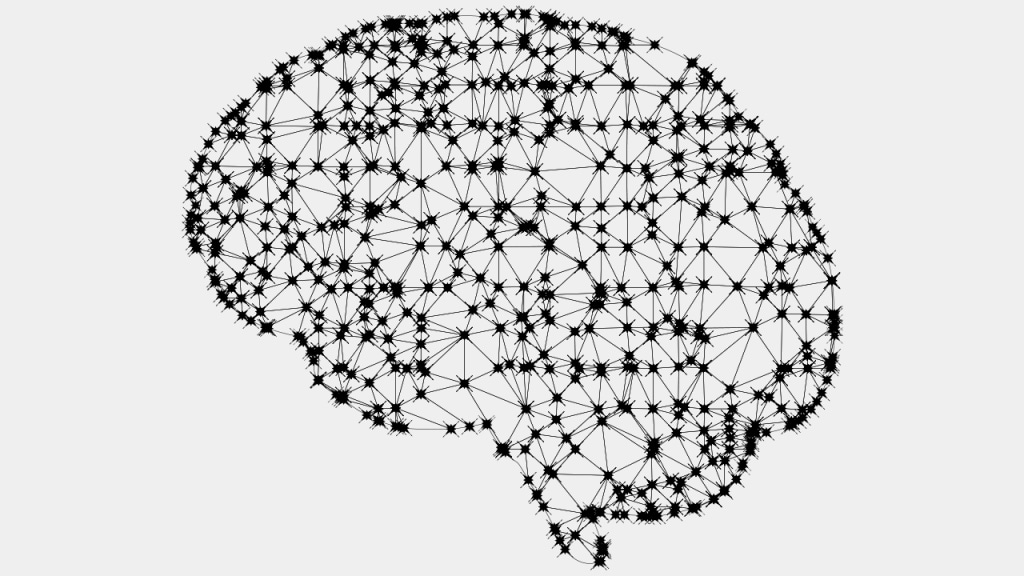
Image Representation of the A;
The trio mapped a complete network of nearly 20,000 proteins in our body, with what we know about how they interact with each other, and ultimately included the nearly 4 million of them. known interactions of these proteins with pharmaceuticals.
This has found patterns of why and how a certain drug causes a protein to behave differently. This gargantuan task was outsourced to Decagon, who used Deep Learning – an AI instrument that crunched complex data and what could be eliminated as counter-intuitive models by people to find any against -Indication possible for a chosen cocktail drug.
The team also explained that Decagon finding these models does not make it absolutely certain, but most of his predictions that they later tested were bang-on. Citing an example of their presentation, the team shared how Decagon predicted muscle inflammation from a combination of atorvastatin (a cholesterol drug) and amlopidine. (a blood pressure medication), which AI had not planned. The team compared similar Decagon predictions with medical literature and found 50% of them confirmed by recently published studies.
While AI is currently addressing predictions of drug pairs, the team is working to expand its capabilities to complex diets. Once modified to make it more user-friendly for non-computer scientists, the team sees tremendous potential for Decagon by helping physicians make better decisions about which drugs to prescribe, and other researchers to find better drugs. combinations for complex diseases
[ad_2]
Source link