[ad_1]
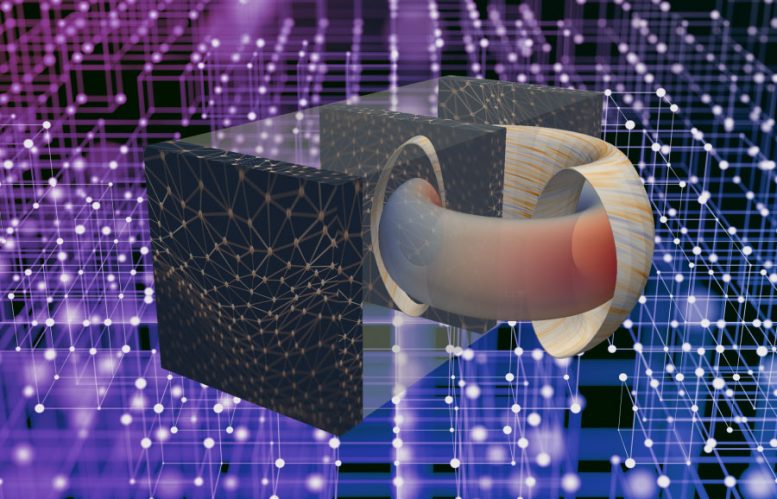
Representation of fusion research on donut-shaped tokamak reinforced by artificial intelligence. (Representing Eliot Feibush / PPPL and Julian Kates-Harbeck / Harvard University)
Artificial intelligence (AI), a branch of computer science that is transforming scientific investigation and industry, could now accelerate the development of a safe, clean and safe fusion energy. practically unlimited for the production of electricity. The Princeton Plasma Plasma Physics Laboratory (PPPL) and Princeton University, where a team of scientists working with a Harvard graduate student are applying for the first time in-depth learning – a powerful new version of the form of Automatic learning of AI – to predict sudden disturbances that can stop the fusion reactions and damage the donut-shaped tokamaks that harbor the reactions.
A promising new chapter in fusion research
"This research opens a promising new chapter in the effort to bring unlimited energy to Earth," said Steve Cowley, Director of PPPL, about the results (link is external), which are reported in the current issue of Nature magazine. . "Artificial intelligence is exploding in all sciences and it is now starting to contribute to the global quest for fusion energy."
Fusion, which drives the sun and the stars, is the fusion of light elements in the form of plasma – the hot and charged state of matter composed of free electrons and atomic nuclei – which generates energy. Scientists seek to replicate the fusion on Earth to obtain an abundant source of energy for the production of electricity.
Access to huge databases provided by two major smelting facilities: the DIII-D National Fusion Facility, managed by General Atomics on behalf of DOE, is critical to demonstrating capacity in-depth learning to predict the disruptions – the sudden loss of containment of plasma particles and energy – has in California, the largest facility in the United States, and the European European Torus (JET ) in the UK, the largest facility in the world, managed by EUROfusion, the European consortium for the development of fusion energy. The support of JET and DIII-D scientists was essential for this work.
Extensive databases have reliably predicted perturbations on tokamaks other than those on which the system was formed – in this case, from the smallest DIII-D to the largest JET. This achievement augurs well for the forecast of disturbances on ITER, a much larger and more powerful tokamak that will have to apply the capabilities acquired on today's fusion facilities.
The deep learning code, called the Recurrent Fusion Neuron Network (FRNN), also opens up possible pathways for controlling and predicting disturbances.
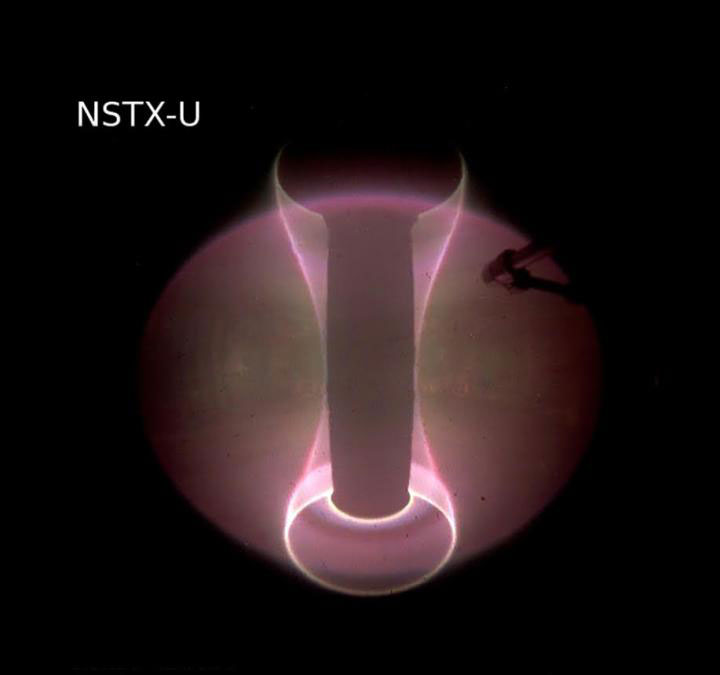
Fast camera photo of a plasma produced by the first campaign NSTX-U operations.
Most intriguing area of scientific growth
"Artificial intelligence is the most intriguing field of scientific growth at the moment, and marrying it to the science of fusion is very exciting," said Bill Tang, senior research physicist at PPPL, co-author of the paper and lecturer with the title of Professor in the Department of Astrophysical Sciences at Princeton University who oversees the AI project. "We have accelerated the ability to accurately predict the most dangerous challenge of clean fusion energy."
Unlike traditional software, which perform the prescribed instructions, deep learning learns from mistakes. Neural networks, layers of interconnected nodes – mathematical algorithms – that are "parameterized" or weighted by the program to give the desired shape to magic are particularly effective. For any given input, the nodes seek to produce a specified output, such as a correct identification of a face or accurate forecasts of a disturbance. The training starts when a node fails to perform this task: the weights automatically adjust for new data until the correct output is obtained.
A key element of deep learning is its ability to capture large data rather than one-dimensional data. For example, although a non-thorough learning software may take into account the temperature of a plasma at a given time, the FRNN examines temperature patterns evolving over time and into the future. ;space. "The ability of in-depth learning methods to harness such complex data makes it an ideal candidate for disturbance prediction," said collaborator Julian Kates-Harbeck, a graduate student in physics at the University. Harvard and a graduate in computer science from the DOE-Office of Science. Fellow who was the main author of the Nature paper and the chief architect of the code.
The formation and operation of neural networks is based on graphics processing units (GPUs), computer chips initially designed to render 3D images. These chips are perfectly suited for in-depth learning applications and are widely used by companies to develop artificial intelligence capabilities such as spoken language comprehension and state observation. roads by autonomous cars.
Kates-Harbeck trained the FRNN code on more than two terabytes (1012) of data collected from JET and DIII-D. After running the software on Princeton University's Tiger Modern Graphics Processor Group, the team placed it on Titan, a supercomputer installed at the Oak Ridge Leadership Computing Facility, a utility utility of the DOE Office of Science. other high performance machines.
A demanding task
Distributing the network on many computers was a demanding task. "The formation of deep neural networks is a computational problem that requires the engagement of high-performance computing clusters," said Alexey Svyatkovskiy, co-author of Nature, who helped convert the algorithms into production code. "We have put a copy of our entire neural network on many processors to achieve extremely efficient parallel processing," he said.
The software has also demonstrated its ability to predict true disturbances within the 30 millisecond time required by ITER, while reducing the number of false alarms. The code is now getting closer to the ITER requirement of 95% correct predictions with less than 3% false alarms. Although the researchers say that only a live experimental operation can demonstrate the benefits of any predictive method, their paper notes that the large archival databases used in the forecasts "cover a wide range of operational scenarios and thus provide significant evidence of force the methods considered in this article. "
From forecast to control
The next step will be to move from forecast to disturbance control. "Rather than predicting and mitigating disturbances at the last moment, we would ideally use future deep learning models to gently move plasma away from areas of instability in order to avoid most disturbances, "said Kates-Harbeck. Michael Zarnstorff, who recently left the position of Deputy Director of Research at the PPPL to become the laboratory's scientific lead, highlighted this step. "Control will be essential for post-ITER tokamaks – where disturbance prevention will be an essential requirement," said Zarnstorff.
To move from accurate predictions based on AI to realistic plasma control will require more discipline. "We will combine in-depth learning with basic physics, based on the basics, on high-performance computers, to focus on realistic control mechanisms in hot plasma," Tang said. "By control, we know which buttons turn on a tokamak to change the conditions to avoid disturbances. That's our goal and that's where we go. "
This work is supported by the DOE Graduate Science Fellowship Program, Department of Energy, DOE Office of Nuclear Science and Security; the Institute of Computational Science and Engineering (PICsiE) at Princeton University; and research and development funds led by laboratories provided by PPPL. The authors would like to thank Bill Wichser and Curt Hillegas of PICSciE for their assistance with high performance compute. Jack Wells at the Oak Ridge Leadership Computing Facility; Satoshi Matsuoka and Rio Yokata at the Tokyo Institute of Technology; and Tom Gibbs at NVIDIA Corp.
Publication: M. D. Boyer, et al., "Real-Time Neutron Beam Injection Modeling on NSTX-U Using Neural Networks", Nuclear Fusion, 2019; doi: 10.1088 / 1741-4326 / ab0762
[ad_2]
Source link