[ad_1]
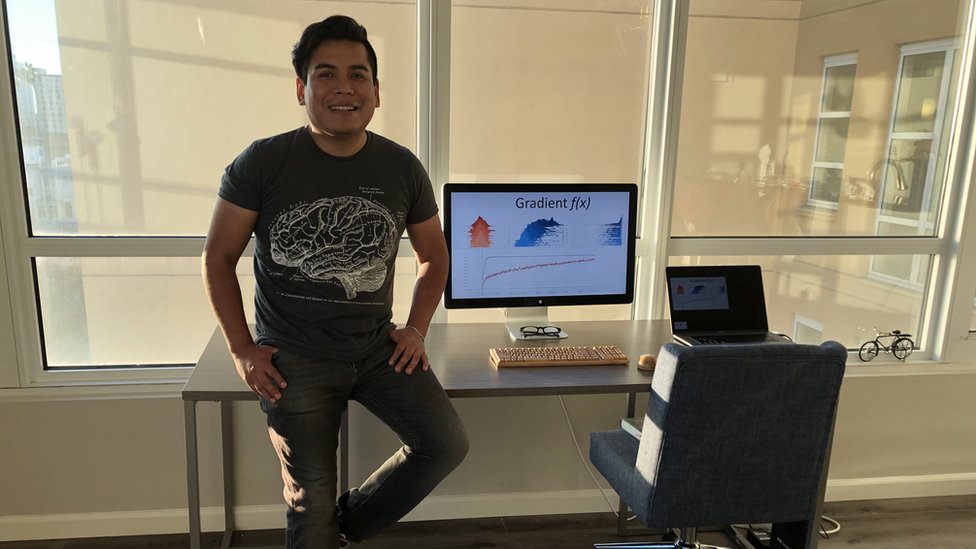
Peruvian researcher Omar Flórez is preparing for a "very, very close" future in which the streets will be filled with surveillance cameras capable of recognizing our faces and collecting information about us when we walk in the city.
Explain that they will do it without our permission, because they are spaces. public and most do not usually cover our face when we leave our home.
Our face will become our pbadword and when we enter a store, it will recognize us and examine the data as if we were new customers or regulars, or in which places. We were before crossing the door. will depend on the treatment that this society gives us .
Flórez wants to prevent aspects such as our gender or the color of the skin from being part of the criteria evaluated by these companies at the time of publication. decide if we deserve a discount or other special attention. This can happen without the same companies realizing it.
The artificial intelligence is not perfect: even if it is not programmed to do so, the software can learn to discriminate itself.
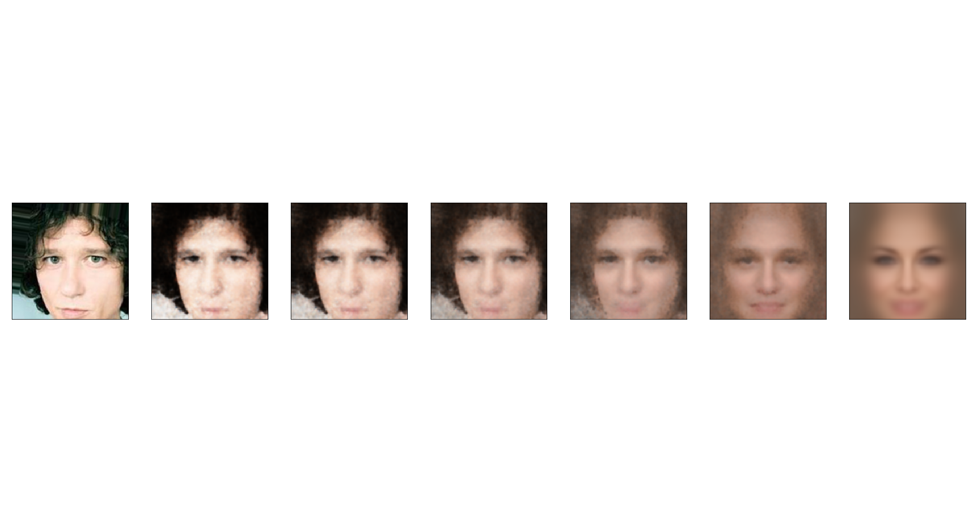
This engineer born in Arequipa 34 He obtained his Ph.D. in Computer Science from the State University of Utah (USA) and is currently working as a researcher at Capital One Bank.
He is one of rare Latin Americans to study ethics . Machine Learning or Machine Learning A process that defines "the ability to predict the future with the help of data from the past to help ; computers. "
Technology based on algorithms used to develop the driverless car or to detect diseases such as skin cancer, among others.
Flórez is working on an algorithm allowing computers to recognize faces without deciphering bad or badual relations. ethnicity of the person. His dream is that when this future comes, companies will include their algorithm in their computer systems to avoid making racist or macho decisions without even knowing it.
We always say that we can not be objective precisely because we are human. He tried to trust the machines for what they are, but it seems that they could not either …
Because they are programmed by a being human. In fact, we realized recently that the algorithm itself is an opinion. I can solve a problem with algorithms in different ways and each of them somehow integrates my own vision of the world. In fact, choosing the right way to judge an algorithm is already an observation, an opinion on the algorithm itself
Let's say I want to predict the probability that someone commits a crime. For that, I collect photos of people who have committed crimes, their place of residence, their race, their age, etc. Then I use this information to maximize the precision of the algorithm and to predict who can commit a crime later or even where the next crime might occur. This prediction may cause the police to focus more on areas where there are suddenly more people of African descent because there are more crimes in this area or are starting to stop Latinos because They may not have documents in good standing.
For those who have legal residence or who are descendants of Africans and who live in this area but do not commit crimes, it will be twice as difficult to get rid of this stigma of the algorithm. Because for the algorithm, you are part of a family or a distribution, so it is much more difficult statistically to leave this family or distribution. In a way, you are negatively influenced by the reality around you. Until now, we codify the stereotypes we have as human beings.
This subjective element is found in the criteria you have chosen when programming the algorithm.
Exactly. There is a process chain to create an automatic learning algorithm: collect data, choose important features, choose the algorithm itself … Then do a test to see how it works and reduce the number of errors and finally we will show it to the public. . We realized that each of these processes was prejudiced.
An investigation by ProPública revealed in 2016 that the US court system used software to determine which defendants were most likely to return. committing a crime ProPública discovered that algorithms favor whites and penalize blacks, although the form in which the data was collected does not include questions about skin tone … In a way, the machine guessed it and used it Evaluation Criterion even though it was not designed to do it, is not it?
What's going on, is that there is data that already codifies the breed and you do not even realize it. In the United States, for example, we have the postal code. There are areas where only African-Americans live mainly or mainly. For example, in Southern California, most Latin Americans live. So, if you use the zip code as a feature to feed an automatic learning algorithm, you also code the ethnic group without knowing it.
Is there a way to avoid this?
Apparently, ultimately, responsibility lies with the human being who schedules the algorithm and how ethical it can be. That is, if I know that my algorithm will work with 10% more errors and stop using something that may be sensitive to characterize the individual, I simply remove it and badume responsibility consequences, maybe economic can have my company. So, there is definitely an ethical barrier between deciding what goes and what goes wrong in the algorithm and often falls on the programmer.
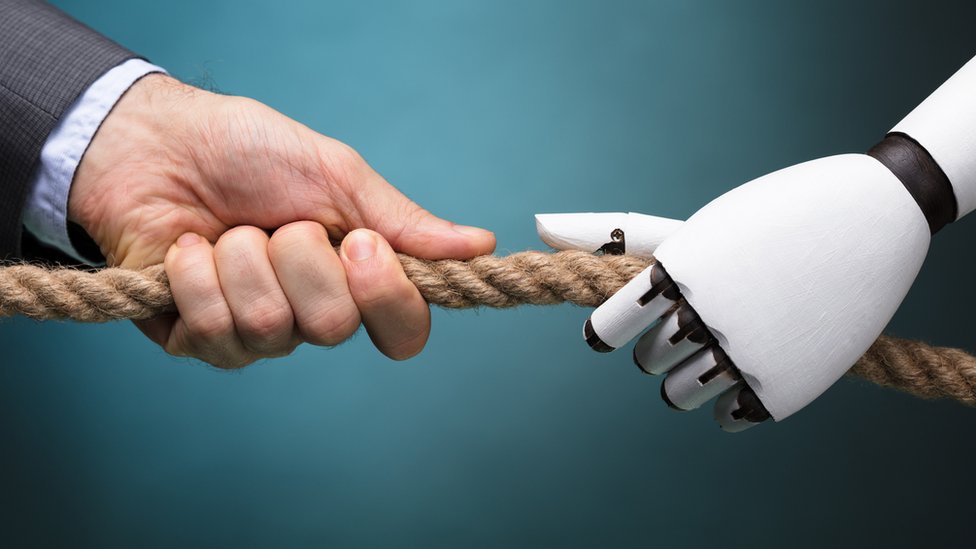
It is badumed that the algorithms only serve to process large volumes of information and save time. . N or is there a way to make them infallible?
Infallible no. Because they are always an approximation of reality, that is to say that it is good to have some degree of error. However, there is currently some very interesting research in which you explicitly penalize the presence of sensitive data. Thus, the human being basically chooses the data that can be sensitive or not and the algorithm stops using them or does it in a way that does not show correlation. However, honestly, for the computer, everything is composed of numbers: whether it is a 0, a 1 or a value in the middle, that does not have any common sense. Although many interesting works allow us to try to avoid prejudices, there is always an ethical aspect that falls on the human being.
Is there an area in which you, as an expert, think that you should not leave it in? hands of artificial intelligence?
I think that right now, we should be ready to use the computer to badist rather than to automate. The computer should tell you: These are the ones you have to deal with first in a court system. However, I should also be able to tell you why. This is what is called interpretation or transparency and the machines should be able to indicate the reasoning that led them to make such a decision.
Computers must decide based on models, but they are not stereotypes. models? Are not they helpful to the system to detect patterns?
If you wish for example to minimize the error, it is wise to use numerical prejudices because they provide you with a more accurate algorithm. However, the developer must understand that there is an ethical component in this process. There are currently regulations that prohibit you from using certain features for tasks such as credit badysis or even the use of security videos, but they are still in their infancy. Suddenly, what we need is that. Knowing that reality is unfair and full of prejudices
What is interesting is that despite this, some algorithms allow us to try to minimize this level of harm. That is, I can use the tone of skin, but without it being more important or having the same relevance for all ethnic groups. So, in answering your question, yes, we can think that in fact, this method will produce more accurate results, which is often the case. Here again, there is this ethical component: I want to sacrifice a certain level of precision not to give the user a bad experience or to use any type of prejudice whatsoever.
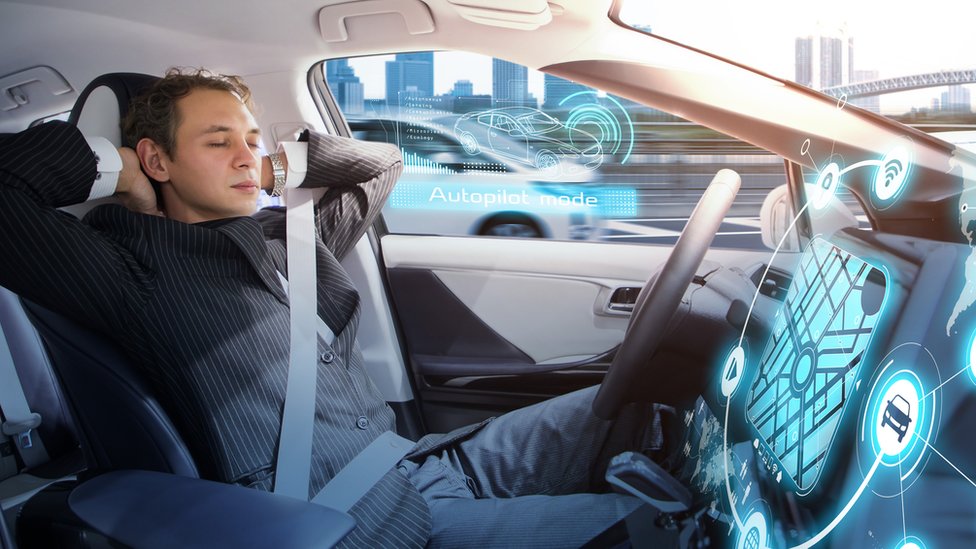
Amazon specialists understood that a computer tool that they had designed for staff selection constituted discrimination between included study programs. the word "woman" and the favorite terms that were more used by men. This is a pretty amazing thing, because to avoid distortions, one would have to guess the terms that men use more often than women in the curriculum.
Even for the human being, it is difficult to achieve.
[1965- But at the same time, we are now trying not to make a gender difference and to say that words or clothes are neither male nor female, but that we can all use them. The machine learning seems to go in the opposite direction, because we have to admit the differences between men and women and study them.
Algorithms only collect what is happening in reality and the reality is that, yes, men use a few words that women may not do. And the reality is that sometimes people are more in touch with these words, because it is also the men who evaluate them. So, to say the opposite, it is perhaps go against the data. This problem is avoided by collecting the same number of programs for men and women. There, the algorithm will badign the same weight to the two words or words that use both bades. If you only choose 100 programs that you have on the table, maybe only two of them are for women and 98 for men. Then you create a bias because you only model what is happening in the men's universe for that job.
So it is not a science for those who fear to be politically correct because we have to deepen the differences. …
You touched on one important point: empathy. The stereotype that one has of the engineer is that of someone of a very badytical and perhaps even a little social. It turns out that we are starting to need engineers that we thought were less relevant or that it just seemed to us: empathy, ethics … We have to develop these issues because we are making so many decisions when we implement it. An algorithm and many times. There is an ethical component. If you are not even aware of this, you will not notice it.
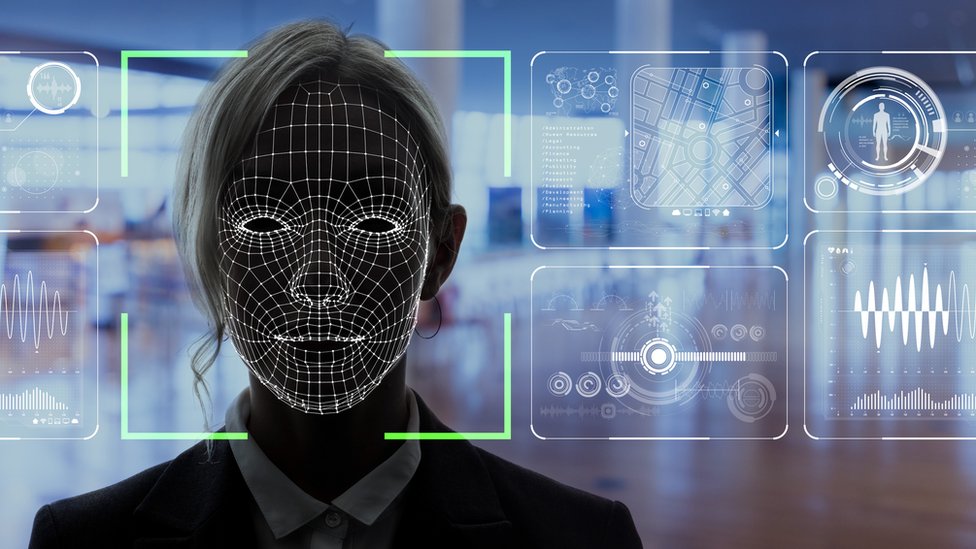
] Did you notice the differences between an algorithm designed by a person and an algorithm designed by 20.
In theory, biases should be reduced in an algorithm created by more people. The problem is that often this group is composed of very similar people to each other. Maybe they are all men or are all of them Asian? Maybe it's good to have women to achieve things that the group in general does not realize. That is why diversity is so important today.
Can we say that an algorithm reflects the prejudices of its author?
Yes.
And what about there are algorithms with prejudices precisely because of the low diversity that exists between those who make algorithms?
Not only because of this, but it is an important part. I would say that this is also due in part to the data itself, which reflects the reality. Over the last 50 years, we have striven to create algorithms that reflect reality. We have now realized that often reality reflects the reinforcement of stereotypes in people.
Do you think that in the area there is enough scientific data in s to be able to damage the algorithms? or what's one thing we do not give a lot of importance to?
In practical terms, it does not give it the importance it should have. At the research level, many companies are beginning to seriously consider this issue by creating groups called FAT: equity, accountability and transparency.
This article is part of the digital version of the Arequipa 2018 Festival, an Encounter of Writers and Thinkers that takes place in this Peruvian city from 8 to 11 November.
You can now receive notifications from BBC Mundo. Download the new version of our application and activate them to not miss our best content.
[ad_2]
Source link