[ad_1]
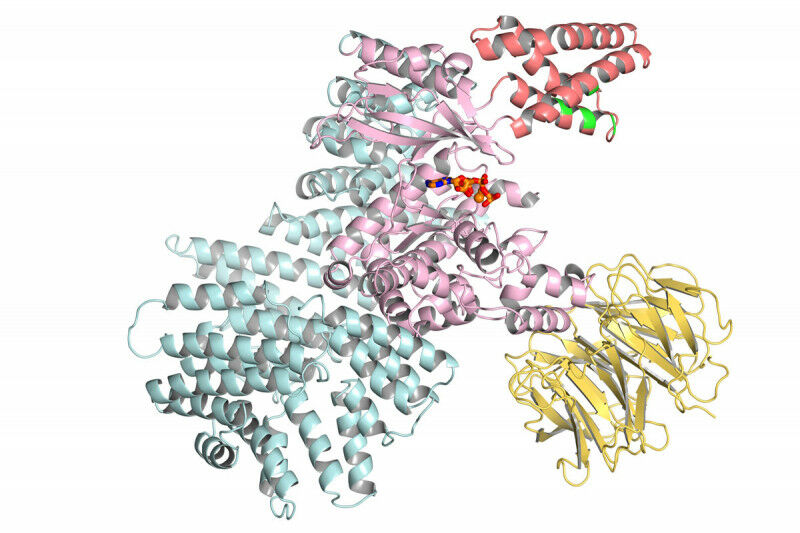
Just a week after Google’s DeepMind AI group finally described its biology efforts in detail, the company publishes an article that explains how it analyzed almost every protein encoded in the human genome and predicted its likely three-dimensional structure, a structure that can be critical. to understand the disease and design treatments. In the very near future, all these structures will be released under a Creative Commons license via the European Institute of Bioinformatics, which already hosts a large database of protein structures.
In a press conference associated with the newspaper’s publication, Demis Hassabis of DeepMind made it clear that the company is not stopping there. In addition to the work described in the article, the company will publish structural predictions for the genomes of 20 major research organisms, from yeast to fruit flies to mice. In total, the database launch will include approximately 350,000 protein structures.
What is there in a structure?
We just described DeepMind’s software last week, so we won’t go into detail here. The effort is an AI-based system formed on the structure of existing proteins that had been determined (often painstakingly) by laboratory experiments. The system uses this training, along with information it obtains from evolutionarily linked protein families, to predict how a protein’s amino acid chain folds in three-dimensional space.
The resulting three-dimensional structure can provide us with essential information about the protein, such as how it interacts with other proteins and chemicals, and where the protein’s chemical reactions take place. Using the structure, researchers can learn how specific mutations, like those that cause genetic diseases, alter the protein’s function. Researchers can also use the structure to design chemicals that can interact with the protein and alter its function, which has led to therapies for various cancers and HIV.
Normally, these structures are determined by isolating the protein, preparing it for imaging, and bombarding it with electrons. These techniques are difficult and time consuming, and they often fail. The article estimates that decades of laboratory work have left us with structural information for only 17% of the complete set of human proteins.
This explains why researchers have also spent decades looking for ways to predict protein structures using only the sequence of amino acids that make them up. But before AlphaFold, the accuracy of the software was not high enough to still be useful.
The human protein collection
DeepMind has not attempted to predict the structure of every protein in the human genome; some are just too big to handle conveniently. (The company set the size limit at 2,700 amino acids, which is unfortunately smaller than a gene that I spent some of my post-doctoral cloning on.) But most proteins are much smaller than that, so the final number is 98.5% genome protein. Some of these proteins should only exist on the basis of the characteristics of DNA sequences in the human genome.
Equally important, AlphaFold includes a confidence estimate that records the likelihood that its predictions are correct. All in all, the software is confident about the location of about 60% of the amino acids it predicted, and it is very confident about just over a third. In other words, the researchers have a confident prediction about most of the structure of 40 percent of human proteins. Obviously, this means that there is a lot of work to be done before we can say that we have good control over all human proteins. But it’s still a parcel more than the 18 percent for which we have actual structures.
There is also a large collection of proteins that are not well represented by existing structures. Those embedded in a cell’s membrane are difficult to isolate and use, so researchers have not resolved many structures of these membrane proteins. But although it has fewer examples in its training data, AlphaFold seems to handle structures quite well.
Where is the system having problems? Many proteins simply don’t form a defined structure – in fact, their function seems to depend on a completely flexible structure in order to function. Obviously, it’s difficult to make precise predictions of a structure here, as these proteins (more generally, sections of proteins) don’t. There are also many proteins that take their structure only when in contact with another protein or chemical. Since AlphaFold does not have this information, there is little it can do.
In general, the DeepMind team found that AlphaFold had very low confidence in their predictions for disordered regions, and they could use this information to identify areas of protein that might be unstructured.
Everything becomes public
In the near future (maybe by the time you read this) all of this data will be available on a dedicated website hosted by the European Institute for Bioinformatics, an organization funded by the European Union that describes itself in part as follows: : “We make global public biological data freely accessible to the scientific community through a range of services and tools. AlphaFold data will be no exception; once the above link is online, anyone can use it to download information on the human protein of their choice.
Or, as mentioned above, the mouse, yeast, or fruit fly version. The 20 organizations that will see their data released are just the start, too. Demis Hassabis of DeepMind said that over the next few months, the team will target every genetic sequence available in DNA databases. By the time this work is completed, over 100 million proteins are expected to have predicted structures. Hassabis concluded his part of the announcement by saying, “We believe this is the most significant contribution AI has made to science to date.” It would be difficult to argue otherwise.
That said, there are still a few issues to be addressed. There will undoubtedly be improvements to the algorithm over time, so it will take a system to handle updating and versioning in the main database. DeepMind also made AlphaFold’s code open source, so there is potential for forks and other complications.
But these problems are concerns for the future. For now, we can all sit back and watch the servers scramble to serve nearly every biologist on the planet who is curious to see if a protein they’re interested in has high-quality structure.
(Unless your humble author, because my protein of choice was too oversized.)
Nature, 2021. DOI: 10.1038 / s41586-021-03828-1 (About DOIs).
[ad_2]
Source link