[ad_1]
An artificially intelligent software could help doctors treat a problem that is literally a pain in the ass: prostate cancer.
A team of radiologists at the University of California at Los Angeles has set up a convolutional neural network to analyze MRI scans of subnational male regions and detect signs of cancer. These types of scans are a much less invasive method than physicians who are delicately interested in collecting tissue for biopsies.
To inspect MRI images, physicians need extensive training and assessments are often based on interpretations. The researchers thought that computer algorithms could perhaps help doctors diagnose the disease.
The neural network, named FocalNet, detects prostate cancer by analyzing the pixels in the MRI to look for lesions in the prostate region. These small areas of damaged tissue are benign or cancerous. One way to check is to compare the cell structure using the Gleason score, a scoring system that estimates the aggressiveness of cancer.
Prostate cancer can be cured when it is caught in its infancy. FocalNet predicts the severity of the disease in patients from their MRI scans by ranking each type in one of six groups. One group contains members with a healthy prostate, no lesions, while the other five groups are associated with different classifications of the Gleason score. A higher score indicates that the disease has progressively worsened and that cancer cells spread more to other parts of the body.
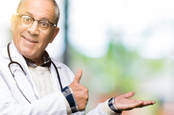
Not so fast, Doctor, FDA would like to check if you are really healthy
READ MORE
Examinations of 417 patients, who had undergone an MRI before prostate cancer surgery, were used to form the CNN. The data contained a total of 728 lesions and the researchers used a Nvidia Titan Xp graphics processing unit with 12 GB of memory to analyze all the numbers. Each 2D scan was first processed as a vector and fed into FocalNet as input, while the outputs were categorized into six different categories.
FocalNet achieved the best results when analyzing patients with the highest risk of prostate cancer. Its accuracy was 80.5% for the most aggressive lesions and 79.2% for the less advanced stages still considered "clinically significant" and 55.6% for the rest of the range. In comparison, three radiologists with at least 10 years of MRI analysis performed better with an accuracy of 83.9% for the most aggressive, 80.7% or clinically significant cases and 61.8% for all the lesions.
This is a comparable comparison with 0.62 false positives per patient for radiologists and software.
Thus, from an oral point of view, human experts beat a neural network as part of a university study that is not used for production. Hopefully this technology will eventually improve with more data.
The research was presented this month at the IEEE International Symposium on Biomedical Imaging in Italy. ®
Sponsored:
Become a leader in pragmatic security
[ad_2]
Source link